Estimation of corn yield thro ugh image treatments obtained by Sentinel 2: case of Las Arenas, Acambay
DOI:
https://doi.org/10.29312/remexca.v16i3.3636Keywords:
biophysical indicators, corn production, vegetation indicesAbstract
Corn (Zea mays L.) is the basis of food and culture in the state of Mexico, so estimating its production to sustain a growing population is a current need. Therefore, images obtained by ESA Copernicus’ Sentinel 2 were used to estimate corn yield in plots in the localities of Las Arenas, Acambay, state of Mexico. The efficiency of various indices and biophysical indicators calculated with information from these images was tested to establish their correlation against the harvest measured in the field. The indices calculated in Sentinel 2 were: NDVI and EVI and the LAI and FAPAR indicators. In this region and under conditions of intense drought, the NDVI calculated in Sentinel 2 had the best predictive ability for corn yield (model fit r2= 0.79). Based on the correlation, the production of 10 randomly selected plots was estimated, demonstrating that, in the range of values between 0.4 and 0.5, NDVI is an excellent predictor of corn harvest under drought conditions, whereas higher NDVI values tend to overestimate yield by up to 1 t ha-1. This information is useful for estimating the harvest and insurance of agricultural production.
Downloads
References
Asrar, G.; Fuchs, M.; Kanemasu, E. T. y Hatfield, J. L. 1984. Estimación de la radicación fotosintéticamente absorbida y el índice de área foliar a partir de la reflectancia espectral del trigo. Agronomy Journal. 76(2):300-306. https://acsess.onlinelibrary.wiley.com/doi/10.2134/agronj1984.00021962007600020029x.
Bolton, D. K. and Friedl, M. A. 2013. Forecasting crop yield using remotely sensed vegetation indices and crop phenology metrics. Agricultural and Forest Meteorology. 173:74-84. Doi.org/10.1016/j. agrformet.2013.01.007.
Chen, J. M. and Black, T. A. 1992. Defining leaf area index for non-flat leaves. Plant, Cell & Environment. 15(4):421-429.
CONAGUA, 2022. Comisión Nacional del Agua. Estaciones meteorológicas automáticas (EMAS). https://smn.conagua.gob.mx/es/observandoeltiempo/estacionesmeteorologicasautomaticasemas.
Champo-Jiménez, O.; España-Boquera, M. L.; Sánchez-Vargas, N.; Cruz-de-León, J.; Lobit, P. y López-Pérez, L. 2014. Construcción de mapas de LAI y fAPAR de la Reserva de la Biósfera Mariposa Monarca y su comparación con mapas globales. CienciaUAT. 8(2):22-31. https://www.redalyc.org/articulo.oa?id=441942931003.
Chi, X.; Yangling, D. and Dai, Z. 2022. Estimation of maize biomass components from Sentinel-1 SAR data using multi-target regressors. IEEE International Geoscience and Remote Sensing Symposium. 1392-1395 pp. Doi:10.1109/IGARSS46834.2022.9884054.
Chuvieco-Salinero, E. 2002. Teledetección ambiental. La observación de la tierra desde el espacio. 1a. Ed. Editorial Ariel. Barcelona, España. 586 p.
CIMMYT. 2012. Centro Internacional de Mejoramiento de Maíz y Trigo. Manual de estimación de rendimiento de maíz. Mexico. El Batán, Estado de México. 16-27 pp.
Cruz-Durán, J. P.; Sánchez-García, A.; Galvis-Spínola, P. y Carrillo-Salazar, J. A. 2011. Índices espectrales en pimiento para el diagnóstico nutrimental de nitrógeno. Terra Latinoamericana. 29(3):259-265.
Doraiswamy, P. C.; Moulin, S.; Cook, W. P. and Stern, A. 2003. Crop yield assessment from remote sensing. Photogramm. Eng. Remote Sens. 69(6):665-674.
Hernández, N.; Soto, F. y Caballero, A. 2009. Modelos de simulación de cultivos: Características y usos. Cultivos Tropicales. 30(1):73-82. http://scielo.sld.cu/scielo.php?script=sci-arttext&pid=S025859362009000100014&lng=es&tlng=es.
Hu, R.; Yan, G.; Mu, X. and Luo, J. 2014. Indirect measurement of leaf area index on the basis of path length distribution. Remote Sensing of Environment. 155:239-247. Doi: 10.1016/j.rse.2014.08.032.
Huang, J.; Wang, H.; Dai, Q. y Han, D. 2014. Analysis of NDVI data for crop identification and yield estimation. IEEE Journal of Selected Topics in Applied Earth Observations and Remote Sensing. 7(11):4374-4384.
Kolotii, A.; Kussul, N.; Shelestov, A.; Skakun, S.; Yailymov, B.; Basarab, R. y Ostapenko, V. 2015. comparación de predictores biofísicos y satélites para predicciones de rendimiento de trigo en Ucrania. Archivos internacionales de fotogrametría, teledetección y ciencias de la información espacial. 39-44 pp.
Lewis, J. E.; Rowland, J. y Nadeau, A. 1998. Estimating maize production in Kenya using NDVI: some statistical considerations. International Journal of Remote Sensing, 19(13):2609-2617.
Manzo-Delgado, L. y Meave, J. A. 2003. La vegetación vista desde el espacio. La fenología foliar a través de la percepción remota. Ciencia. 54(3):18-28. https://www.amc.edu.mx/revistaciencia/images/revista/54-3/vegetacion-vista-espacio.pdf.
Ovando, G.; de la Casa, A.; Díaz, G.; Díaz, P.; Bressanini, L. y Miranda, C. 2021. Desempeño de diferentes índices de vegetación de Sentinel-2A para estimar el rendimiento de soja en agricultura de precisión. Agriscientia. 38(2):1-12.
Prasad, A. K.; Chai, L.; Sigh, R. P. and Kafatos, M. 2006. Crop yield estimation model for Iowa using remote sensing surface parameters. International Journal of Applied Earth Observation and Geoinformation. 8(1):26-33.
QGIS 2024. Geographic Information System. Open-Source Geospatial Foundation Project. https://qgis.org.
Qin, H.; Wang, C.; Zhao, K. and Xi, X. 2018. Estimación de la fracción de radiación fotosintéticamente activa absorbida (fPAR) en los canopies de maíz utilizando datos LiDAR e imágenes hiperespectrales. Plos one. 13(5):e0197510. Doi.org/10.1371/journal.pone.0197510.
Soria-Ruiz, J.; Fernández-Ordóñez, Y. y Granados-Ramirez, R. 2004. Metodología para la predicción del rendimiento del maíz utilizando datos satelitales de teledetección en el Centro de México. Investigaciones Geográficas. 55:61-78.
Vega-Araya, M. y Alvarado-Barrantes, R. 2019. Análisis de las series de tiempo de variables biofísicas para cuatro ecorregiones de Guanacaste, Costa Rica. Revista de Ciencias Ambientales. 53(2):60-96. Doi: https://doi.org/10.15359/rca.53-2.
Weiss, M.; Troufleau, D.; Baret, F.; Chauki, H.; Prévot, L.; Olioso, A.; Bruguier, N. y Brisson, N. 2001. Coupling canopy functioning and radiative transfer models for remote sensing data assimilation. Agric. For. Meteorol. 108(2):113-128.
Xiuliang, J.; Zhenhai, L.; Haikuan, F.; Zhibin, R. y Shaokun, L. 2019. Deep neural network algorithm for estimating maize biomass based on simulated Sentinel 2A vegetation indices and leaf area index. KeAi Chinese Roots Global Impact. 8(1):87-97.
Xu, C.; Ding, Y.; Zheng, X.; Wang, Y.; Zhang, R.; Zhang, H.; Dai, Z. and Xie, Q. A. 2022. Comprehensive comparison of machine learning and feature selection methods for maize biomass estimation using Sentinel-1 SAR, Sentinel-2 Vegetation Indices, and Biophysical Variables. Remote Sensing. 14:4083. Doi.org/10.3390/rs14164083.
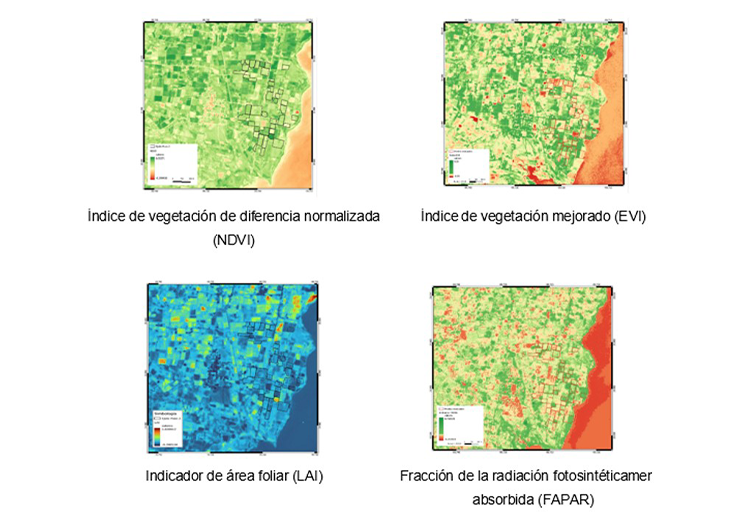
Published
How to Cite
Issue
Section
License
Copyright (c) 2025 Revista Mexicana de Ciencias Agrícolas

This work is licensed under a Creative Commons Attribution-NonCommercial 4.0 International License.
The authors who publish in Revista Mexicana de Ciencias Agrícolas accept the following conditions:
In accordance with copyright laws, Revista Mexicana de Ciencias Agrícolas recognizes and respects the authors’ moral right and ownership of property rights which will be transferred to the journal for dissemination in open access. Invariably, all the authors have to sign a letter of transfer of property rights and of originality of the article to Instituto Nacional de Investigaciones Forestales, Agrícolas y Pecuarias (INIFAP) [National Institute of Forestry, Agricultural and Livestock Research]. The author(s) must pay a fee for the reception of articles before proceeding to editorial review.
All the texts published by Revista Mexicana de Ciencias Agrícolas —with no exception— are distributed under a Creative Commons License Attribution-NonCommercial 4.0 International (CC BY-NC 4.0), which allows third parties to use the publication as long as the work’s authorship and its first publication in this journal are mentioned.
The author(s) can enter into independent and additional contractual agreements for the nonexclusive distribution of the version of the article published in Revista Mexicana de Ciencias Agrícolas (for example include it into an institutional repository or publish it in a book) as long as it is clearly and explicitly indicated that the work was published for the first time in Revista Mexicana de Ciencias Agrícolas.
For all the above, the authors shall send the Letter-transfer of Property Rights for the first publication duly filled in and signed by the author(s). This form must be sent as a PDF file to: revista_atm@yahoo.com.mx; cienciasagricola@inifap.gob.mx; remexca2017@gmail.
This work is licensed under a Creative Commons Attribution-Noncommercial 4.0 International license.