Rendimiento y estabilidad de genotipos de soya para el trópico de México
DOI:
https://doi.org/10.29312/remexca.v12i8.2267Palabras clave:
biplot, genotipo ideal, rendimiento relativoResumen
Actualmente el cambio climático obliga a los fitomejoradores a desarrollar genotipos adaptados a mega-ambientes, lo que garantiza la correcta producción del cultivo. El objetivo de este estudio fue determinar el potencial en rendimiento de grano y estabilidad de genotipos de soya. Para estos fines, se evaluaron 15 genotipos de soya (siete variedades y ocho líneas experimentales) a través de cuatro años (2014, 2015, 2016 y 2017), en un diseño látice cuadrado 5x5 con tres repeticiones. El análisis de varianza reveló diferencias significativas en años, genotipos y en la interacción genotipos por años. Siendo la fuente de variación años, la que tuvo mayor impacto en el rendimiento con un 84.3%, seguido de la interacción genotipos por años (10%) y de genotipos (5.6%). Asimismo, las condiciones del 2014 fueron más propicias para que los genotipos tuvieran un mayor rendimiento. Con relación a los genotipos, el G15, G7, G5 y G2 presentaron los mayores valores de rendimiento a través de los cuatro años. En cuanto a estabilidad y rendimiento el método de rendimiento relativo y la representación Biplot GGE coincidieron en que los genotipos que poseen estas dos características son G7 y G2. Por otro lado, se formaron dos mega-ambientes siendo el genotipo G15 el ganador respecto a su desempeño en el primer mega-ambiente donde estuvieron incluidos los años 2015, 2016 y 2017, de la misma forma el genotipo G5 fue el ganador en el segundo mega-ambiente constituido por el año 2014. Los dos métodos al complementarse explicaron mayormente la variación fenotípica del rendimiento.
Descargas
Citas
Bhartiya, A.; Aditya, J. P.; Kumari, V.; Kishore, N.; Purwar, J. P.; Agrawal, A. and Kant, L. 2017. GGE biplot & AMMI analysis of yield stability in multi-environment trial of soybean [Glycine max (L.) Merrill] genotypes under rainfed condition of north western Himalayan hills. J. Anim. Plant Sci. 27(1):227-238.
Bhartiya, A.; Singh, K.; Aditya, J. P.; Puspendra and Gupta, M. 2014. Residual relative heterosis and heterobeltiosis for different agro morphological traits as selection index in early segregating generation of soybean [Glycine max (L.) Merrill] crosses. Soybean res. 12(1):28-35.
Brar, K. S.; Singh, P.; Mittal, V. P.; Singh, P.; Jakhar, M. L.; Yadav, Y.; Sharma, M. M.; Shekhawat, U. S. and Kumar, C. 2010. GGE biplot analysis for visualization of mean performance and stability for seed yield in taramira at diverse locations in India. J. Oilseed Brassica. 1(2):66-74.
Casanoves, F.; Baldessari, J. and Balzarini, M. 2005. Evaluation of multienvironment trials of peanut cultivars. Crop Sci. 45(1):18-26. doi:10.2135/cropsci2005.0018. DOI: https://doi.org/10.2135/cropsci2005.0018
Dia, M.; Wehner, T. C.; Hassell, R.; Price, D. S.; Boyhan, G. E.; Olson, S.; King, S.; Davis, A. R. and Tolla, G. E. 2016. Genotype environment interaction and stability analysis for watermelon fruit yield in the United States. Crop Sci. 56(4):1645-1661. doi:10.2135/ cropsci2015.10.0625. DOI: https://doi.org/10.2135/cropsci2015.10.0625
Ebdon, J. S. and Gauch, H. G. 2002. Additive main effect and multiplicative interaction analysis of national turfgrass performance trials. Crop Sci. 42(2):497-506. doi:10.2135/cropsci200 2.0489.
Eberhart, S. T. and Russell, W. A. 1966. Stability parameters for comparing varieties. Crop Sci. 6(1):36-40. doi: 10.2135/cropsci196 6.0011183X000600010011x. DOI: https://doi.org/10.2135/cropsci1966.0011183X000600010011x
Farshadfar, E.; Rashidi, M.; Jowkar, M. M. and Zali, H. 2013. GGE Biplot analysis of genotype environment interaction in chickpea genotypes. Eur. J. Exp. Biol. 3(1):417-423.
Finlay, K. W. and Wilkinson, G. N. 1963. The analysis of adaptation in a plant breeding programme. Aust. J. Agric. Res. 14(6):742-754. doi: 10.1071/AR9630742. DOI: https://doi.org/10.1071/AR9630742
Gauch, H. G.; Piepho, H. P. and Annicchiarico, P. 2008. Statistical analysis of yield trials by AMMI and GGE: Further considerations. Crop Sci. 48(3):866-889. doi:10.2135/cropsci2007. 09.0513. DOI: https://doi.org/10.2135/cropsci2007.09.0513
Haider, Z.; Akhter, M.; Mahmood, A. and Khan, R. A. R. 2017. Comparison of GGE biplot and AMMI analysis of multi-environment trial (MET) data to assess adaptability and stability of rice genotypes. African J. Agric. Res. 12(51):3542-3548. doi:10.5897/AJAR2017.12528. DOI: https://doi.org/10.5897/AJAR2017.12528
Imtiaz, M.; Malhotra, R. S.; Singh, M. and Arslan, S. 2013. Identifying high yielding, stable chickpea genotypes for spring sowing: specific adaptation to locations and sowing seasons in the Mediterranean region. Crop Sci. 53(4):1472-1480. doi:10.2135/cropsci2012.10.0589. DOI: https://doi.org/10.2135/cropsci2012.10.0589
Kang, M. S. 1993. Simultaneous selection for yield and stability in crop performance trials: Consequences for growers. Agron. J. 85(3):754-757. DOI: https://doi.org/10.2134/agronj1993.00021962008500030042x
Kang, M. S. and Gorman, D. P. 1989. Genotype × environment interaction in maize. Agron. J. 81(4):662-664. doi: 10.2134/agron j1989.00021962008100040020x. DOI: https://doi.org/10.2134/agronj1989.00021962008100040020x
Kumudini, S. 2010. Soybean growth and development. The soybean: botany, production and uses. (Ed.) by Guriq Singh. Ludhiana, India. 23 p. DOI: https://doi.org/10.1079/9781845936440.0048
López-Castañeda, C. 2011. Variación en rendimiento de grano, biomasa y número de granos en cebada bajo tres condiciones de humedad del suelo. Trop. Subtrop. Agroecosyst. 14(3):907-918.
López, S. E.; Acosta, G. J. A.; Tosquy, V. O. H.; Salinas, P. R. A.; Sánchez, G. B. M.; Rosales, S. R.; González, R. C.; Moreno, G. T.; Villar, S. B. Cortinas; E. H. M. y Zandate, H. R. 2011. Estabilidad de rendimiento en genotipos mesoamericanos de frijol de grano en México. Rev. Mex. Cienc. Agríc. 2(1):29-40.
Lu’quez, J. E.; Aguirreza’bal, L. A. N.; Aguero, M. E. and Pereyra, V. R. 2002. Stability and adaptability of cultivars in non- balanced yield trials: comparison of methods for selecting ‘high oleic’ sunflower hybrids for grain yield and quality. J. Agron. Crop Sci. 188(4):225-234. doi:10.1046/j.1439-037X.2002.00562.x. DOI: https://doi.org/10.1046/j.1439-037X.2002.00562.x
Magari, R. and Kang, M. S. 1993. Genotype selection via a new yield stability statistic in maize yield trials. Euphytica. 70(1-2):105-111. doi: 10.1007/BF00029647. DOI: https://doi.org/10.1007/BF00029647
Maldonado, M. N. 2017. Soya de temporal y riego para el sur de Tamaulipas ciclo P-V. Agenda Técnica Agrícola Tamaulipas. Instituto Nacional de Investigaciones Forestales, Agrícolas y Pecuarias (INIFAP). Ciudad de México. 92-105 pp.
Mustapha, M. and Bakari, H. R. 2014. Statistical evaluation of genotype by environment interactions for grain yield in millet (Penniisetum glaucum (L.) R. Br.). Int. J. Eng. Sci. 3(9):7-16.
Pecina, Q. V.; Maldonado, H. L.; Maldonado, M. N.; Simpson, J.; Martínez, V. O. y Gil, V. K. C. 2005. Diversidad genética en soya del Trópico Húmedo de México determinada con marcadores AFLP. Rev. Fitotec. Mex. 28(1):63-69.
R. 2020. R: A Language and environment for statistical computing. R foundation for statistical computing. Vienna, Austria. Retrieved from http://www.r-project.org/.
Rimieri, P. 2017. La diversidad y la variabilidad genéticas: dos conceptos diferentes asociados al germoplasma y al mejoramiento genético vegetal. BAG. J. Basic Appl. Gen. 28(2):7-13.
SAS. 2014. SAS: Business analytics and business intelligence software. NC, USA: SAS Inst. Retrieved from http://www.sas.com/en-us/home.html.
Shukla, S.; Mishra, B. K.; Mishra, R.; Siddiqui, A.; Pandey, R. and Rastogi, A. 2015. Comparative study for stability and adaptability through different models in developed high thebaine lines of opium poppy (Papaver somniferum L.). Ind. Crops prod. 74:875-886. doi:10.1016/j.indcrop.2015.05.076. DOI: https://doi.org/10.1016/j.indcrop.2015.05.076
Smith, A. B.; Cullis, B. R. and Thompson, R. 2005. The analysis of crop cultivar breeding and evaluation trials: An overview of current mixed model approaches. J. Agric. Sci. 143(06):449-462. doi: 10.1017/S0021859605005587. DOI: https://doi.org/10.1017/S0021859605005587
Vaezi, B.; Pour-Aboughadareh, A.; Mohammadi, R.; Armion, M.; Mehraban, A.; Hossein-Pour, T. and Dorii, M. 2017. GGE biplot and AMMI analysis of barley yield performance in Iran. Cereal res. Commun. 45(3):500-511. doi:10.1556/0806.45.2017.019. DOI: https://doi.org/10.1556/0806.45.2017.019
Yan, W. 2001. GGE biplot a Windows application for graphical analysis of multienvironment trial data and other types of two-way data. Agron. J. 93(5):1111-1118. DOI: https://doi.org/10.2134/agronj2001.9351111x
Yan, W. and Tinker, N. A. 2005. An integrated biplot analysis system for displaying, interpreting and exploring genotype environment interaction. Crop Sci. 45(3):1004-1016. doi:10.2135/cropsci2004.0076. DOI: https://doi.org/10.2135/cropsci2004.0076
Yan, W. and Kang, M. S. 2003. GGE biplot analysis: a graphical tool for breeders, geneticists, and agronomists. CRC Press. Boca Raton, London, New York, Washington, D.C. retrieved from https://content.taylorfrancis.com/books/download?dac=c2009-0-09571-8&isbn=978 0429122729&format=googlepreviewpdf. 263 p.
Yan, W. 2002. Singular-value partitioning in biplot analysis of multienvironment trial data. Agron. J. 94(5):990-996. doi:10.2134/AGRONJ2002.9900. DOI: https://doi.org/10.2134/agronj2002.0990
Yan, W.; Hunt, L. A.; Sheng, Q. and Szlavnics, Z. 2000. Cultivar evaluation and mega-environment investigation based on the GGE biplot. Crop Sci. 40(3):597-605. doi:10.2135/cropsci2000. 403597x. DOI: https://doi.org/10.2135/cropsci2000.403597x
Yan, W.; Kang, M. S.; Ma, B.; Woods, S. and Cornelius, P. L. 2007. GGE biplot vs. AMMI analysis of genotype-by-environment data. Crop sci. 47(2):643-655. doi:10.2135/cropsci2006. 06.0374. DOI: https://doi.org/10.2135/cropsci2006.06.0374
Yan, W. and Tinker, N. A. 2006. Biplot analysis of multi-environment trial data: principles and applications. Can. J. Plant Sci. 86(3):623-645. doi:10.4141/P05-169. DOI: https://doi.org/10.4141/P05-169
Yang, R. C.; Crossa, J.; Cornelius, P. L. and Burgueño, J. 2009. Biplot analysis of genotype environment interaction: proceed with caution. Crop Sci. 49(5):1564-1576. doi:10.2135/cropsci2008.11.0665. DOI: https://doi.org/10.2135/cropsci2008.11.0665
Yau, S. K. and Hamblin, J. 1994. Relative yield as a measure of entry performance in variable environments. Crop Sci. 34(3):813-817. doi:10.2135/cropsci1994.0011183X0034000 30038x. DOI: https://doi.org/10.2135/cropsci1994.0011183X003400030038x
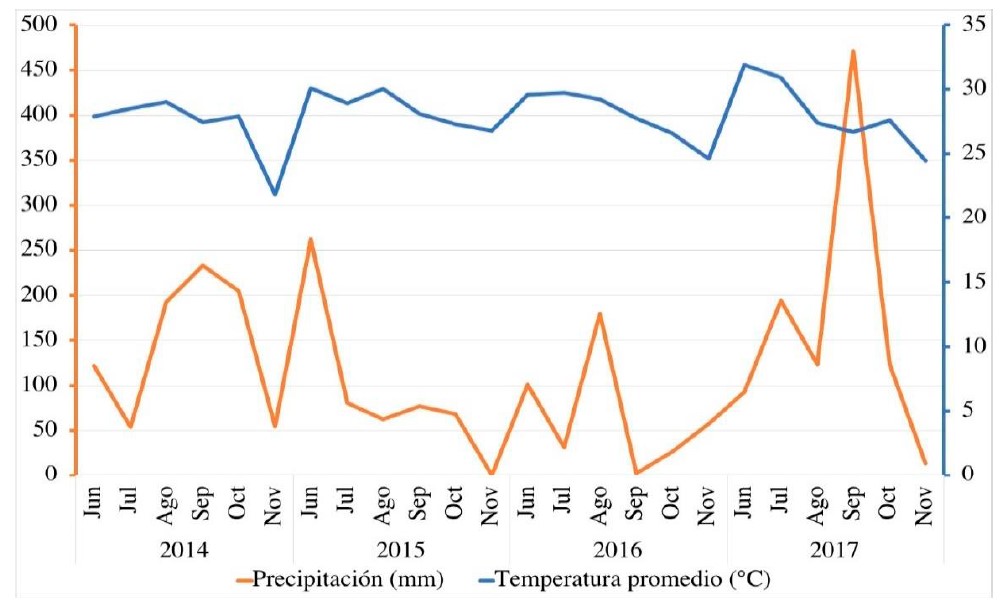
Descargas
Publicado
Cómo citar
Número
Sección
Licencia
Derechos de autor 2021 Revista Mexicana de Ciencias Agrícolas

Esta obra está bajo una licencia internacional Creative Commons Atribución-NoComercial 4.0.
Los autores(as) que publiquen en Revista Mexicana de Ciencias Agrícolas aceptan las siguientes condiciones:
De acuerdo con la legislación de derechos de autor, Revista Mexicana de Ciencias Agrícolas reconoce y respeta el derecho moral de los autores(as), así como la titularidad del derecho patrimonial, el cual será cedido a la revista para su difusión en acceso abierto.
Los autores(as) deben de pagar una cuota por recepción de artículos antes de pasar por dictamen editorial. En caso de que la colaboración sea aceptada, el autor debe de parar la traducción de su texto al inglés.
Todos los textos publicados por Revista Mexicana de Ciencias Agrícolas -sin excepción- se distribuyen amparados bajo la licencia Creative Commons 4.0 atribución-no comercial (CC BY-NC 4.0 internacional), que permite a terceros utilizar lo publicado siempre que mencionen la autoría del trabajo y a la primera publicación en esta revista.
Los autores/as pueden realizar otros acuerdos contractuales independientes y adicionales para la distribución no exclusiva de la versión del artículo publicado en Revista Mexicana de Ciencias Agrícolas (por ejemplo incluirlo en un repositorio institucional o darlo a conocer en otros medios en papel o electrónicos) siempre que indique clara y explícitamente que el trabajo se publicó por primera vez en Revista Mexicana de Ciencias Agrícolas.
Para todo lo anterior, los autores(as) deben remitir el formato de carta-cesión de la propiedad de los derechos de la primera publicación debidamente requisitado y firmado por los autores(as). Este formato debe ser remitido en archivo PDF al correo: revista_atm@yahoo.com.mx; revistaagricola@inifap.gob.mx.
Esta obra está bajo una licencia de Creative Commons Reconocimiento-No Comercial 4.0 Internacional.