Predictors of the price of white corn in Jalisco and Michoacán
DOI:
https://doi.org/10.29312/remexca.v13i2.2740Keywords:
arima, forecast, seriesAbstract
Corn is one of the most important products in the world due to its nutritional qualities related to human, animal consumption and industrial use. A predictor of corn price behavior is useful for producers and marketers in making decisions. A price indicator is provided in international stock exchanges; however, in Mexico there is no stock exchange that provides an adequate signal about the future behavior of white corn prices in Mexico. In this research, an analysis of white corn prices in Michoacán and Jalisco was carried out, using autoregressive integrated moving average (ARIMA) models with the aim of providing a predictor of white corn prices. Two models were built for each series and point estimates were made. The predictive capacity of the models was evaluated using the mean absolute percentage error, the root-mean-square error and Theil’s U. The results showed that the price of corn in Michoacán and Jalisco can be predicted by its past values with an AR (1) model and an MA (2) model. It was concluded that these models provide a predictor for corn prices and constitute a useful tool in planning and making decisions regarding the process of production, commercialization and related products.
Downloads
References
Akaike, H. 1974. A new look at the statistical model identification, IEEE transactions on automatic control. 19(6):716-723. Doi:10.1109/TAC.1974.1100705.
Adebiyi, A.; Adewumi, O. A. and Ayo, C. K. 2014. Stock price prediction using the Arima model. UKSim-AMSS 16th International conference on computer modelling and simulation. IEEE. Reino Unido. IEEE. 2014(1):106-112. Doi: 10.1109/UKSim.2014.67.
F. 2013. Uso de un modelo univariado de series de tiempo para la predicción del comportamiento de la producción de carne de cerdo en Baja California, México. México. Rev. Cien. 5(23):403-409.
Bowerman, B. L.; O’Connell, R. T. y Koehler, A. B. 2007. Pronósticos, series de tiempo y regresión un enfoque aplicado México. Cengage learning. 4a (Ed.). México, DF. 717 p.
Box, G.; Jenkins, G.; Reinsel, G. and Ljung, G. 2016. Time series analysis forecasting and control. Wiley. 5a (Ed.). New Yersey. 709 p.
Box, G. and Pierce, D. 1970. Distribution of residual autocorrelations in autoregressive-integrated moving average time series models. J. Am. Stat. Assoc. 65(332):1509-1526.
Contreras, A.; Atziry, C.; Martínez, J. L. y Sánchez, D. 2016. Análisis de series de tiempo en el pronóstico de la demanda de almacenamiento de productos perecederos. España. Estudios Gerenciales. 32(141):387-96. https://doi.org/10.1016/j.estger.2016.11.002.
Chu, K. 1978. Short-run forecasting of commodity prices: an application of autoregressive moving average models. International Monetary Fund. 25(1):90-111. https://doi.org/10.2307/ 3866657.
Cryer, D. J. and Sik, K. 2008. Time series analysis with applicatios. In: Springer, R. 2a (Ed.). New York. 502 p. https://doi.org/10.1007/BF00746534.
Delgadillo-Ruiz, O.; Ramírez-Moreno, P. P.; Leos-Rodríguez, J. A.; Salas, J. M. y Valdez-Cepeda, R. D. 2016. Pronósticos y series de tiempo de rendimientos de granos básicos en México. México. Acta Universitaria Miltidisc. Scientif. J. 3(26):23-32. https://doi.org/10.15174/ au.2016.882.
Enders, W. 2015. Applied econometrics time series. Wiley. 4a (Ed.). United States of America. 498 p.
FIRA. 2016. fideicomisos instituidos en relación con la agricultura. Panorama agroalimentario 2016.
FIRA. 2019. fideicomisos instituidos en relación con la agricultura. Panorama agroalimentario 2019. Gujarati, D. N. and Porter, D. C. 2010. Econometría. McGraw-Hill Publishing Educacion. 5a (Ed.). México. 946 p.
Greene, W. H. 2012. Econometric analysis. Pearson. 7a (Ed.). New York University. 1241 p.
Jamal, F.; Ezzine, L. and Aman, Z.; El Moussami, H. and Lachhab, A. 2018. Forecasting of demand using ARIMA model. Inter. J. Eng. Bus. Manag. 10(1):1-9. Doi: 10.1177/18479790 18808673.
Ljung, G. and Box, G. 1978. On a measure of a lack of fit in time series models. Biometrika. 65(2):297-303. Doi:10.1093/biomet/65.2.297.
Luis-Rojas, S.; García-Sánchez, R.; García-Mata, R.; Arana-Coronado, O. A. and Gonzáles-Estrada, A. 2019. Metodología Box-Jenkins para pronosticar los precios de huevo blanco pagados al productor en México. Agrociencia. 6(53):911-925.
Makridakis, S.; Wheelwright, S. C. and Hyndman, R. J. 1997. Forecasting, methods and applications. Jhon Wiley and Sons. 3a (Ed). EU. 656 p.
Marroquín, G. y Chalita, L. E. 2010. Aplicación de la metodología Box-Jenkins para pronóstico de precios en jitomate. México. Rev. Mex. Cienc. Agríc. 4(2):573-577. http://www.redalyc.org/articulo.oa?id=263119723008.
Ortiz A. y Montiel, A. N. 2017. Transmisión de precios futuros de maíz del chicago board of trade al mercado spot mexicano. México. Contaduría y Administración. (62):924-940. https://doi.org/10.1016/j.cya.2016.01.004.
Pankratz, A. 1983. Forecasting with univariate Box-Jenkins models concepts and cases. Jhon Wiley and Sons. EU. 588 p.
Quintana, L. y Mendoza, M. A. 2010. Econometría aplicada utilizando R. Universidad Nacional Autónoma de México (UNAM). México. 358 p.
SAGARPA. 2017. Secretaría de Agricultura Ganadería Desarrollo Rural Pesca y Alimentación. Planeación agrícola nacional 2017-2030. México, DF.
Sánchez- López, E.; Barreras-Serrano, A.; Pérez-Linares, C.; Figueroa-Saavedra, F. y Olivas-Valdez J. A. 2013. Aplicación de un modelo ARIMA para pronosticar la producción de leche de bovino en baja california, México. Trop. Subtrop. Agroecosys. 3(16):315-324.
SIAP. 2020. Servicio de Información Agroalimentaria y Pesquera. https://nube.siap.gob.mx/ cierreagricola/.
SIAP. 2019. Servicio de Información Agroalimentaria y Pesquera. Panorama agroalimentario 2019. México, DF.
SIAP. 2012. Servicio de Información Agroalimentaria y Pesquera. Situación actual y perspectivas del maíz en México 1996-2012. http://www.campomexicano.gob.mx/portal-siap/ Integracion/EstadisticaDerivada/ComercioExterior/Estudios/Perspectivas/maiz96-12.pdf.
SNIIM. 2018. Sistema Nacional de Información e Integración de Mercados. Secretaría de Economía. http://www.economia-sniim.gob.mx/nuevo/.
Schwarz, G. 1978. Estimating the dimension of a model. Annals of statistics. 6(2):461-464. MR 468014. doi:10.1214/aos/1176344136.
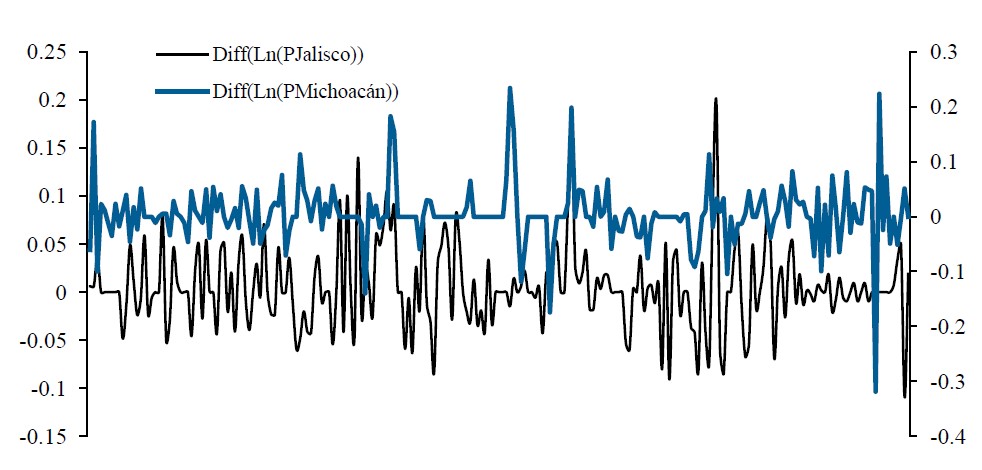
Published
How to Cite
Issue
Section
License
Copyright (c) 2022 Revista Mexicana de Ciencias Agrícolas

This work is licensed under a Creative Commons Attribution-NonCommercial 4.0 International License.
The authors who publish in Revista Mexicana de Ciencias Agrícolas accept the following conditions:
In accordance with copyright laws, Revista Mexicana de Ciencias Agrícolas recognizes and respects the authors’ moral right and ownership of property rights which will be transferred to the journal for dissemination in open access. Invariably, all the authors have to sign a letter of transfer of property rights and of originality of the article to Instituto Nacional de Investigaciones Forestales, Agrícolas y Pecuarias (INIFAP) [National Institute of Forestry, Agricultural and Livestock Research]. The author(s) must pay a fee for the reception of articles before proceeding to editorial review.
All the texts published by Revista Mexicana de Ciencias Agrícolas —with no exception— are distributed under a Creative Commons License Attribution-NonCommercial 4.0 International (CC BY-NC 4.0), which allows third parties to use the publication as long as the work’s authorship and its first publication in this journal are mentioned.
The author(s) can enter into independent and additional contractual agreements for the nonexclusive distribution of the version of the article published in Revista Mexicana de Ciencias Agrícolas (for example include it into an institutional repository or publish it in a book) as long as it is clearly and explicitly indicated that the work was published for the first time in Revista Mexicana de Ciencias Agrícolas.
For all the above, the authors shall send the Letter-transfer of Property Rights for the first publication duly filled in and signed by the author(s). This form must be sent as a PDF file to: revista_atm@yahoo.com.mx; cienciasagricola@inifap.gob.mx; remexca2017@gmail.
This work is licensed under a Creative Commons Attribution-Noncommercial 4.0 International license.