Genotype-environment interaction of yield in yellow corn hybrids, using AMMI and SREG
DOI:
https://doi.org/10.29312/remexca.v13i7.3070Keywords:
Zea mays, biplot graph, double and trilinear hybrids, stability and adaptabilityAbstract
It is indispensable for corn (Zea mays L.) plant breeding programs to select homogeneous materials, with high yield and with stable agronomic attributes; also, that they have a good adaptability in contrasting environments. The objective of the work was to evaluate the stability and genotype-environment interaction of the yield of 36 hard yellow corn hybrids, evaluated in seven environments of Peru, during 2016-2018, these materials were analyzed using the AMMI (additive main effects and multiplicative interaction) and SREG (site regression) models. The design used in each experiment was a 6×6 lattice with three repetitions, and the response variable was grain yield. A combined analysis of variance was performed, in which statistical differences between them (p≤ 0.05) were detected, then the Tukey mean test (p≤ 0.05) was applied, finally the AMMI and SREG models were run and the biplot graphs of each statistical model were obtained. Of the interaction between PC1 and PC2, AMMI explained 45.5% and 15.3%, respectively, and SREG with 59.8% and 12.2%, for the same components. The trilinear hybrids Dk-5005 and AG-01 outperformed the double-cross hybrids. The AMMI model detected the existing GE interaction in grain yield, and the SREG accurately grouped the assessment sites into six mega-environments. The three environments of La Molina and that of Huánuco identified the two hybrids (Dk-5005 and AG-01) with the highest grain yield (11.524 and 11.359 t ha-1, respectively).
Downloads
References
Camargo, B. I.; Quiros, M. E. y Gordon, M. R. 2011. Identificación de mega‑ambientes para potenciar el uso de genotipos superiores de arroz en Panamá. Pesquisa Agropecuária Brasileira. 46(9):1061-1069. Doi.org/10.1590/S0100-204X2011000900013.
Castillo, D.; Matus, I.; Pozo, A.; Madariaga, R. and Mellado, M. 2012. Adaptability and genotype × environment interaction of spring wheat cultivars in Chile using regression analysis, AMMI, and SREG. Chilean J. Agric. Res. 72(2):167-174. Doi.org/10.4067/S0718-583920 12000200001.
Chura, C. J. y Huanuqueño, C. E. H. 2014. Comportamiento de ocho poblaciones de maíz amarillo (Zea mays L.) en cruzas con un probador. Anales Científicos. 76(1):78-86. Doi.org/10.21704/ac.v76i1.767.
Crossa, J.; Vargas, M.; Cossani, C. M.; Alvarado, G.; Burgueño, J.; Mathews, K. L. and Reynolds, M. P. 2015. Evaluation and interpretation of interactions. Agron. J. 107(2):736-747. Doi.org/10.2134/agronj2012.0491.
Dia, M.; Wehner, T. C. and Arellano, C. 2016. Analysis of genotype × environment interaction using SAS programming. Agron. J. 108(5):1838-1852. Doi.org/10.2134/agronj2016.02.0085.
Eberhart, S. A. and Russel, W. A. 1966. Stability parameters for comparing varieties. Crop Sci. 6(1):36-40. Doi.org/10.2135/cropsci1966.0011183X000600010011x. Farias, F. J. C.; Carvalho, P. L.; Silva, F. J. L. and Teodoro, E. P. 2016. Biplot analysis of phenotypic stability in upland cotton genotypes in Mato Grosso. Genet. Mol. Res. 15(2):1-10. Doi.org/10.4238/gmr.15028009. Fayeun, L. S.; Alake, C. G. and Akinlolu, O. A. 2018. GGE biplot analysis of fluted pumpkin (Telfairia occidentalis) landraces evaluated for marketable leaf yield in Southwest Nigeria. J. Saudi Soc. Agric. Sci. 17(4):416-423. Doi.org/10.1016/j.jssas.2016.10.001. Fritsche, N. R.; Vieira, M. G.; Oliveira, D. R. and Namorato, S. H. 2010. Factor analysis and SREG GGE biplot for the genotype × environment interaction stratification in maize. Ciência Rural. 40(5):1044-1048. Doi.org/10.1590/S0103-84782010000500007.
Gómez, M. Y.; Boicet, F. T.; Tornés, O. N. y Meriño, H. Y. 2018. Interacción genotipo ambiente de cuatro variedades de tomate en la provincia Granma. Rev. Centro Agrícola. 45(2):21-28.
Kandus, M.; Almorza, D.; Boggio R. R. and Salerno, J. C. 2010. Statistical models for evaluating the genotype-environment interaction in maize (Zea mays L.). International J. Exp. Bot. 79(1):39-46. Doi:10.32604/phyton.2010.79.039.
Ledesma, R. L.; Solís, M. E.; Suaste, F. M. P.; Rodríguez, C. J. F. y Cruz, G. M. L. 2012. Análisis GGE biplot del rendimiento de trigo (Triticum spp.) con riego normal y restringido en el Bajío, México. Agrociencia. 46(2):119-131.
López, M. F.; Chura, C. J. y García, P. G. 2019. Interacción genotipo por ambiente del rendimiento de maíz amarillo en híbridos trilineales, Perú. Rev. Mex. Cienc. Agríc. 10(4):859-872. Doi.org/10.29312/remexca. v10i4.1696.
Lozano, R. Á.; Santacruz, V. A.; San-Vicente, G. F.; Crossa, J.; Burgueño, J. y Molina, G. J. D. 2015. Modelación de la interacción genotipo ambiente en rendimiento de híbridos de maíz blanco en ambientes múltiples. Rev. Fitotec. Mex. 38(4):337-347. Doi.org/10.35196/rfm. 2015.4.337.
Manrique, C. P. A. 1997. El maíz en el Perú. Consejo Nacional de Ciencia y Tecnología. (CONCYTEC). Lima, Perú. 362 p.
MINAGRI. 2020. Ministerio de Agricultura y Riego. Sistema de información de cultivos de maíz amarillo duro. Lima, Perú. http://sissic.minagri.gob.pe/sissic.
Ndhlela, T.; Herselman, L.; Magorokosho, C.; Setimela, P.; Mutimaamba, C. and Labuschagne, M. 2014. Genotype environment interaction of maize grain yield using AMMI biplots. Crop Science. 54(5):1992-1999. Doi.org/10.2135/cropsci2013.07.0448.
Neisse, A. C.; Kirch, L. J. and Hongyu, K. 2018. AMMI and GGE biplot for genotype × environment interaction: a medoid-based hierarchical cluster analysis approach for high-dimensional data. Biometrical Letters. 55(2):97-121. Doi.org/10.2478/bile-2018-0008.
SAS Institute. 2012. User’s Guide of SAS. SAS Institute Inc. Cary, North Carolina, USA. 550 p.
SENAMHI. 2020. Servicio Nacional de Meteorología e Hidrología del Perú. Mapa climático nacional. Ministerio del Ambiente. Lima, Perú. https://www.senamhi.gob.pe/?&p=mapa-climatico-del-peru.
Tadeo, R M.; Espinosa, C. A.; Guzmán, M. R.; Turrent, F. A.; Zaragoza, E. J. y Virgen, V. J. 2015. Productividad de híbridos varietales de maíz de grano amarillo para Valles Altos de México. Agron. Mesoam. 26(1):65-72. Doi.org/10.15517/am.v26i1.16921.
Vallejo, C. F. A. y Estrada, S. E. I. 2002. Interacción genotipo-ambiente. In: Vallejo, C. F. A. y Estrada, S. E. I. (Ed.). Mejoramiento genético de plantas. Universidad Nacional de Colombia. Palmira, Colombia. 189-202 pp.
Vargas, E. E. A.; Vargas, S. J. E. y Baena, G. D. 2016. Análisis de estabilidad y adaptabilidad de híbridos de maíz de alta calidad proteica en diferentes zonas agroecológicas de Colombia. Acta Agronómica. 65(1):72-79. Doi.org/10.15446/acag.v65n1.43417.
Yan, W.; Pageau, D.; Frégeau, R. J.; Lajeunesse, J.; Goulet, J.; Durand, J. and Marois, D. 2011. Oat mega-environments and test-locations in Quebec. Canadian J. Plant Sci. 91(4):643-649. Doi.org/10.4141/cjps10139.
Yan, W.; Frégeau, R. J.; Pageau, D. and Martin, R. 2016. Genotype-by-environment interaction and trait associations in two genetic populations of oat. Crop Sci. 56(3):1136-1145. Doi.org/10.2135/cropsci2015.11.0678.
Yang, R. C.; Crossa, J.; Cornelius, P. L. and Burgueño, J. 2009. Biplot analysis of genotype environment interaction: proceed with caution. Crop Sci. 49(5):1564-1576. Doi.org/10.2135/cropsci2008.11.0665.
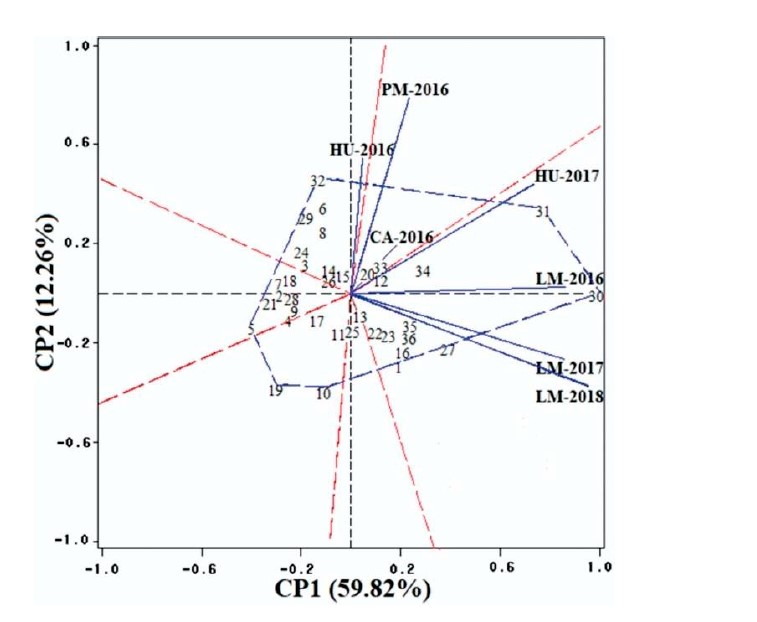
Published
How to Cite
Issue
Section
License
Copyright (c) 2022 Revista Mexicana de Ciencias Agrícolas

This work is licensed under a Creative Commons Attribution-NonCommercial 4.0 International License.
The authors who publish in Revista Mexicana de Ciencias Agrícolas accept the following conditions:
In accordance with copyright laws, Revista Mexicana de Ciencias Agrícolas recognizes and respects the authors’ moral right and ownership of property rights which will be transferred to the journal for dissemination in open access. Invariably, all the authors have to sign a letter of transfer of property rights and of originality of the article to Instituto Nacional de Investigaciones Forestales, Agrícolas y Pecuarias (INIFAP) [National Institute of Forestry, Agricultural and Livestock Research]. The author(s) must pay a fee for the reception of articles before proceeding to editorial review.
All the texts published by Revista Mexicana de Ciencias Agrícolas —with no exception— are distributed under a Creative Commons License Attribution-NonCommercial 4.0 International (CC BY-NC 4.0), which allows third parties to use the publication as long as the work’s authorship and its first publication in this journal are mentioned.
The author(s) can enter into independent and additional contractual agreements for the nonexclusive distribution of the version of the article published in Revista Mexicana de Ciencias Agrícolas (for example include it into an institutional repository or publish it in a book) as long as it is clearly and explicitly indicated that the work was published for the first time in Revista Mexicana de Ciencias Agrícolas.
For all the above, the authors shall send the Letter-transfer of Property Rights for the first publication duly filled in and signed by the author(s). This form must be sent as a PDF file to: revista_atm@yahoo.com.mx; cienciasagricola@inifap.gob.mx; remexca2017@gmail.
This work is licensed under a Creative Commons Attribution-Noncommercial 4.0 International license.