Mathematical modeling and simulation: a tool for crop protection
DOI:
https://doi.org/10.29312/remexca.v13i6.2922Keywords:
computation applied to plant health, decision making, disease managementAbstract
The consequences of climate change on crops are a cause for concern, as they cannot adapt quickly to new pathogenic threats. This article reviews the global use of mathematical models in plant health during the 2000-2020 period, through Scopus and Google Scholar. The objective of this study is to show modeling and simulation as an option in crop protection against meteorological risks or variations that occur due to global warming. Mathematical modeling is not an end in itself but a tool that helps in decision making for the sustainable management of crops. The prediction of the risk of occurrence of a pest or disease favors the reduction of the use of pesticides, thus reducing economic losses and damage to the environment. On the other hand, the simulation or numerical solution of mathematical models allows the exploration of hypotheses, it is an invaluable means in the research, since it allows exploring possible future scenarios and finding options of management systems that will be necessary at some point. In Mexico, it is necessary to carry out research for the generation and implementation of mathematical models that allow a lower cost of production and less negative impact on human health and the environment.
Downloads
References
Achicanoy, L. H. 2000. Descripción cuantitativa de las epidemias de las plantas. Rev. Facultad Nacional Agrícola de Medellín. 1(53): 941-968.
APS. 2018. American Phytopathological Society. Simulation Modeling in Botanical Epidemiology and Crop Loss Analysis. Encontrado. https://www.apsnet.org/edcenter/advanced/topics/ BotanicalEpidemiology/Pages/SimulationModels.aspx.
Aragón, G. A.; Guillen, S. D.; Juárez, L. P. y Alía, T. I. 2018. Interacción geográfica del nicho ecológico de Phyllophaga ravida1 y dos cultivos agrícolas en México. Geographic Interaction of the Phyllophaga ravida1 Ecological Niche and Two Crops in Mexico. Southwestern Entomologist.
Boudon, F.; Pradal, C.; Cokelaer, T.; Prusinkisrewicz, P. and Godin, C. 2012. “L-py: an l-system simulation framework for modeling plant development based on a dynamic language,” Frontiers in Plant Science. 3(76). DOI: https://doi.org/10.3389/fpls.2012.00076
Bouman, B. A. M.; Van Keulen, H.; Van Laar, H. H. and Rabbinge, R. 1996. The ‘School of de Wit’crop growth simulation models: a pedigree and historical overview. Agricultural Systems. 52(2-3):171-198. DOI: https://doi.org/10.1016/0308-521X(96)00011-X
Cabello, T. y Carreño, R. 2002. Modelos logísticos aplicados a la fenología de noctuidos plagas en el sur de España (Lep. Noctuidae). Ministerio de Agricultura, Pesca y Alimentación, Madrid, España.
Campbell, C. L. y Madden, L. V. 1990. Introduction to plant disease epidemiology. Wiley, New York. 532 p.
Dagatti, C. V. y Cristina, B. V. 2015. Ajuste de modelo fenológico para predecir el comportamiento de Lobesia botrana (Lepidoptera: Tortricidae) en un viñedo de Mendoza, Argentina. Revista de la Sociedad Entomológica Argentina. 74(3-4):117-122.
De Wit, C. T. and de Vries, F. P. 1982. L’analyse des systemes de production primaire. In la productivite des paturages Saheliens Pudoc. 918:20-23.
De Wolf, E. D. and Isard, S. A. 2007. Disease cycle approach to plant disease prediction. Annual Review of Phytopathology. 45(9):1-18. DOI: https://doi.org/10.1146/annurev.phyto.44.070505.143329
Durán, P. E.; Téliz, O. D.; Pedroza, S. A.; Mora, A. A.; Ávila, Q. G. D. y González, H. H. 2017. Modelo de pronóstico para el control de la antracnosis del aguacate en Michoacán, México. Colegio de Postgraduados. Fitosanidad-Fitopatología. Tesis de Doctorado. 73 p.
Fernandes, J. X. y Pavan, W. X. 2011. Sistemas de predicción para enfermedades em cereales de inverno: fusariosis y brusone. In: Embrapa Trigo-Artigo em anais de congresso (ALICE). In: Pereyra, S.; Ackermann, MD de; Germán, S.; Cabrera, K. (Ed.). Manejo de enfermedades en trigo y cebada. Montevideo: INIA. 37-39 pp.
Forrester, J. W. 1971. Principles of systems. Pegasus communications, Inc. Waltham, MA. USA. 392 p.
Fransz, H. G. 1974. The Functional Response to Prey Density in An Acarine System. Simulation Monographs. Pudoc, Wageningen. The Netherlands. 143 p.
Freitez, J. A.; Ablan, M. y Gómez, C. 2009. Propuesta de modelos predictivos del brote de la Sigatoka Negra para las plantaciones de plátano al sur del Lago de Maracaibo, Venezuela. Rev. Científica UDO Agrícola. 9(1):191-198.
García, G. C. G.; López, C. J.; Nava, T. M. E.; Villanueva, J. J. A. y Vera, G. J. 2006. Modelo de predicción de riesgo de daño de la mosca pinta Aeneolamia postica (Walker) Fennah (Hemiptera: Cercopidae). Neotropical Entomology. 35:677-688. DOI: https://doi.org/10.1590/S1519-566X2006000500017
Gámez, M.; Carreño, R.; Ándujar, A. S.; Barranco, P. y Cabello, T. 2000. Modelos matemáticos de depredador-presa en cultivos hortícolas en invernadero en el sudeste de la Península Ibérica. Bol San Veg Plagas. 26:665-672.
Garrett, K. A.; Forbes, G. A.; Gómez, L.; Gonzales, M. A.; Gray, M.; Skelsey, P. y Sparks, A. H. 2013. Cambio climático, enfermedades de las plantas e insectos plaga. In: Jiménez, Z. E. (Coord.). Cambio climático y adaptación en el altiplano boliviano. La Paz (Bolivia). CIDES-UMSA SANREM CRSP Universidad de Missouri Universidad Mayor de San Andres; Fundacion PROINPA; Universidad de la Cordillera; Universidad Nacional Agraria La Molina (UNALM).
Gómez, G.; Suárez, M.; Figueroa, M.; Rivero, T. y Hernández, A. 2002. Pronóstico del tizón tardío (Phytophthora infestans (MONT.) de BARY) de la papa en Cuba. II. evaluación de la efectividad del modelo naumova modificado. Fitosanidad. 6(2):35-39.
Gutiérrez, H. O. y García, L. V. 2019. La dimensión geográfica de las invasiones biológicas en el Antropoceno: el caso de Xylella fastidiosa.
Guzmán, F. A.W.; Alatorre, R. R.; Pérez, D. F. y Nolasco, Q. X. 1999. Grados día de desarrollo de Sphenarium purpurascens (Charpentier)(Orthoptera: Pyrgomorphidae) y su susceptibilidad A Beauviera bassiana (Bals.) Vuill. (Deuteromycotina: Hyphomycetes bajo condiciones de campo. Tesis de maestría. Colegio de Postgraduados, Campus Montecillo, Instituto de Fitosanidad, Especialidad en Entomología y Acarología.
Hanan, J.; Prusinkiewicz, P.; Zalucki, M. and Skirvin, D. 2002. Simulation of insect movement with respect to plant architecture and morphogenesis. Computers and Electronics in Agriculture. 35(2-3):255-269. DOI: https://doi.org/10.1016/S0168-1699(02)00022-4
Hernández, D. M. G.; Rojas, M. R. I.; Rivera, P. A.; Zavaleta, M. E.; Ochoa, M. D. L. and Carrillo, S. J. A. 2020. Evaluation of Zebra Chip Using Image Analysis. American Journal of Potato Research. 97(6):586-595.
Hernández, R. E.; Vera, G. J.; Ramírez, V. G.; Pérez, E. S.; López, C. J.; Bautista, M. N. y Pinto, V. M. 2009. Pronóstico de la fluctuación poblacional del minador de la hoja de crisantemo Liriomyza huidobrensis Blanchard (Diptera: Agromyzidae), mediante modelos de series de tiempo. Acta Zoológica Mexicana. 25(1):21-32. DOI: https://doi.org/10.21829/azm.2009.251588
Hernández, Z. M. I.; Quijano, C. J. A.; Yáñez, L. R.; Ocampo, V. R.V.; Torres, P. I.; Guevara, G. R.G. and Castro, R. A. E. 2013. Dynamic simulation model of central American locust Schistocerca piceifrons (Orthoptera: Acrididae). Florida Entomologist. 96(4):1274-1283. DOI: https://doi.org/10.1653/024.096.0405
Hui, J. L.; Er-da, T.; Wheeler, A.; Challinor, P. and Shuai, J. 2013. climate change modelling and its roles to chinese crops yield. J. Int. Agric. 12(5):892-902. DOI: https://doi.org/10.1016/S2095-3119(13)60307-X
INIFAP, 2021. http://www.inifap-nortecentro.gob.mx/. http://clima.inifap.gob.mx/lnmysr.
Jarvis, P. J. 2000. Ecological principles and environmental issues. Prentice-Hall, Nueva York, EEUU.
Jacobo, C. J.; Mora, A. G.; Ramírez, L. M.; Vera, G. J.; Pinto, V. X.; López, C. J. y Aceves, N. L. 2005. Caracterización cuantitativa de la diapausa de palomilla de la manzana Cydia pomonella L. en Cuauhtémoc, Chihuahua, México. Agrociencia. 39(2):221-229.
Kramer, K. 1996. Phenology and growth of European trees in relation to climate change. PhD Thesis. Wageningen University, The Netherlands. 210 p. ISBN 905485 464/2.
Kropff, M. J. 1988. Modelling the effects of weeds on crop production. Weed Research. 28:465-471. DOI: https://doi.org/10.1111/j.1365-3180.1988.tb00829.x
Lindenmayer, A. 1968. Mathematical models for cellular interactions in development I. Filaments with one-sided inputs. Journal of Theoretical Biology. 18(3):280-299. DOI: https://doi.org/10.1016/0022-5193(68)90079-9
López, C. I. L. 2014. Metodología de modelación matemática dinámica de ambientes agrícolas controlados: avances y retos. Universidad Autónoma Chapingo.
López, C. I. L.; Duran, P. E.; Salazar, M. R. y Fitz, R. E. 2022a. Modelo dinámico depredador-presa para Franklinothrips y Scirtothrips en aguacate: simulación y análisis de sensibilidad. In: información, estabilidad y dinámica en los modelos económicos. Asociación Mexicana de Investigación Interdisciplinaria. Pérez, S. F.; Figueroa, H. E.; Salazar, M. R.; Sepúlveda, J. D.; Escamilla, G. P. y Jiménez, G. M. (Comps). ASMIIA AC. Ciudad de México, México. ISBN: 978-607-99921-2-5. 27-42 pp.
López, C. I. L.; Duran, P. E.; Salazar, M. R.; Fitz, R. E. y Sosa, C. J. O. 2021. Dynamic modeling of thrips population in avocado trees. Computación y sistemas.
López, C. I. L.; Duran, P. E.; Fitz, R. E.; Salazar, M. and Rojano, A. A. 2022b. Predator-prey dynamic model for Franklinothrips and Scirtothrips in avocado trees (Persea americana Mill.): simulation and sensitivity analyses.
Magarey, D. R.; Fowler, A. G.; Borchert, M. D.; Sutton, B. T.; Colunga, G. M. and Simpson, A. J. 2007. NAPPFAST: An Internet System for the Weather-Based Mapping of Plant Pathogens. Plant Disease. 91(4):336-345. DOI: https://doi.org/10.1094/PDIS-91-4-0336
Mahalanabis, A. K. 1982. Introductory System Engineering. Wiley, New York. 3-4 pp.
Maloy, O. C. 1993. Plant Disease Control. Wiley, New York. 351-356 pp.
Martínez, A. P. F. y Vargas, H. A. 2016. Modelo dinámico adaptativo para la gestión del agua en el medio urbano. Tecnología y Ciencias del Agua. 7(4):139-154.
Miranda, C. I.; Hernández, O. D.; Hernández, A. Y.; Martínez, C. B. y Rodríguez, H. M. G. 2016. Modelación de la interacción Meloidogyne incognita (Kofoid y White) Chitwood Trichoderma asperellum Samuels, Lieckfeldt & Nirenberg en garbanzo (Cicer arietinum L.). Revista Protección Vegetal. 31(3):194-200.
Mora, A. G.; Acevedo, S. G.; Calderón, E. G.; Flores, S. J.; Domínguez, M. S.; Baker, P. X. y González, G. R. 2014. Consideraciones epidemiológicas del cambio climático en la Fitosanidad de cultivos tropicales. Rev. Mexicana de Fitopatología. 32(2):147-167.
Moschini, R. C.; Bombelli, E. C.; Wright, E. R.; López, M. V.; Perez, C. H. I.; Carmona, J. D. y Rivera, M. C. 2014. Ajuste de modelos logísticos a la tasa de incremento de severidad de manchas foliares ocasionadas por Alternaria tenuissima en arándano= Logistic models adjustment to growth rate of severity of blueberry leaf spot caused by Alternaria tenuissima. Asociación Argentina de Horticultura.
Noriega, N. J. L.; Salazar, M. R. y López, C. I. L. 2021. Revisión: modelos de crecimiento y rendimiento de maíz en escenarios de cambio climático. Rev. Mex. Cienc. Agríc. 12(1):127-140. DOI: https://doi.org/10.29312/remexca.v12i1.2552
Pohlheim, H. and Heißner, A. 1996. Optimal Control of Greenhouse Climate using Genetic Algorithms. in MENDEL’96-2nd International Conference on Genetic Algorithms. Technical University of Brno, Czech Republik. 112-119 pp.
Prusinkiewicz, P. 2021. Algorithmic Botany, Website of the Biological Modeling and Visualization research group in the Department of Computer Science at the University of Calgary. http://algorithmicbotany.org/.
Quishpe, D. N. T. 2020. Modelo de nicho ecológico para predecir la distribución potencial de Antracnosis (Colletotrichum acutatum) en el cultivo de chocho (Lupinus mutabilis Sweet) en Ecuador. (Bachelor’s thesis, Ecuador, Latacunga: Universidad Técnica de Cotopaxi UTC.
Rabbinge, R.; Ward, S. A. and Van Laar, H. H. 1989. Simulation and systems management in crop protection, PUDOC, Wagenigen. 420 p.
Rebellón, J. D. S.; Rodríguez, D. A. L. y Arias, D. G. 2020. Estudio bioinformático del gen CRN8 en Phytophthora infestans causante de la enfermedad tizón tardío en plantas. Microciencia. 9:57-81.
Rocha, C. C. E. y Leal, L. D. D. 2020. Modelo para la detección de la enfermedad Xanthomonas campestris en hojas de judía aplicando algoritmos de optimización genéticos y de gradiente.
Romero, C. T.; López, P. P. A.; Ramírez, L. M. y Cuervo, P. J. A. 2016. Modelado cinético del micoparasitismo por Trichoderma harzianum contra Cladosporium cladosporioides aislado de frutos de cacao (Theobroma cacao). Chilean Journal of Agricultural & Animal Sciences. 32(1):32-45. DOI: https://doi.org/10.4067/S0719-38902016000100004
Rongier, G.; Collon, P.; Renard, P. 2017. Stochastic simulation of channelized sedimentary bodies using a constrained L-system. Computers & Geosciences. 105(2017):158-168. ISSN: 0098-3004. DOI: https://doi.org/10.1016/j.cageo.2017.05.006
Rossing, W. A. H. 1993. On damage, uncertainty, and risk in supervised control: aphids and brown rust in winter wheat as an example. PhD Thesis, Wageningen University, The Netherlands. 202 p. ISBN 90-5485-137-6.
Ruiz, G. A. 2009. Modelos para simulación y control del clima de un invernadero con ventilación natural. Tesis de Maestría en Ingeniería. Universidad Autónoma Chapingo. Chapingo, México. 126 p.
Ruiz, G. A. 2020. La modelación del crecimiento de cultivos: conceptos, aplicaciones y retos. Serie de Seminarios Virtuales 2020. Colegio Mexicano de Ingenieros en Irrigación (C0MEII). México. 30 p.
Scopus. 2022. http://www.scopus.com.
Samano, A. L. L. 2019. Nicho ecológico potencial de ocho especies de Rhagoletis (díptera, tephritidae) de importancia agrícola para México.
Savary, S. and Willocquet, L. 2014. Simulation modeling in botanical epidemiology and crop loss analysis. Plant Health Instructor. 147 pp.
SIAFEG, 2010. http://www.siafeg.com/siafeg/siafeg.htm.
Steduto, P. 2006. Biomasa Water-Productivity. Comparing the growth-engines of crop models. 1-16 p. In: WUEMED Training course. “Integrated approaches to improve drought tolerance in crops”. Department of Agroenvironmental Sciences and Technology, University of Bologna. Bologna.
Taylor, N. 2003. Review of the use of models in informing disease control policy development and adjustment. School of Agriculture, Policy and Development.
Téliz, O. D. y Mora, A. A. 2007. El aguacate y su manejo integrado. Mundiprensa. México. 319 p.
Thornley, J. H. M. and France, J. 2007. Mathematical models in agriculture: quantitative methods for the plant, animal, and ecological sciences. CABI. DOI: https://doi.org/10.1079/9780851990101.0000
Van Ittersum, M. K.; Leffelaar, P. A.; Van Keulen, H.; Kropff, M. J.; Bastiaans, L. y Goudriaan, J. 2003. On approaches and applications of the Wageningen crop models. Eur. J. Agron. 18(3-4):201-234. DOI: https://doi.org/10.1016/S1161-0301(02)00106-5
Van Kraalingen, D. W. G.; Rappoldt, C. y Van Laar, H. H. 2003. The Fortran simulation translator, a simulation language. Eur J Agron. 18(3-4):359-361. DOI: https://doi.org/10.1016/S1161-0301(02)00131-4
Van Straten, G. X. 2012. Systems dynamics for bioengineers. Lectures Notes. University of Chapingo, Chapingo, México. 146 p.
Vidal, D. C. I. y Ortega, F. S. 2001. Validación de un modelo de predicción de la enfermedad pudrición gris de la vid, causada por el hongo Botrytis cinerea, en un viñedo de la séptima región (Doctoral dissertation, Universidad de Talca. Chile.
Vilchez, P. C. R. 2021. Potenciales efectos del cambio climático sobre la distribución del hongo (Moniliophthora roreri Cif & Par) en el cultivo de cacao (Theobroma cacao) en Ecuador (Bachelor’s thesis, Quevedo: UTEQ).
Voinov, A. 2008. Systems science and modeling for ecological economics.
Yáñez, L. R.; Vázquez, O. A.; Arreguín, C. J. H.; Soria, R. J. y Quijano, C. J. Á. 2019. Sistema de alerta contra el gusano cogollero Spodoptera frugiperda (JE Smith) (Insecta: Lepidoptera: Noctuidae). Rev. Mex. Cienc. Agríc. 10(2):405-416. DOI: https://doi.org/10.29312/remexca.v10i2.803
Zadoks, J. C. and Rabbinge, R. 1985. Modelling to a purpose. In: Advances in plant pathology, mathematical modelling of crop diseases. CA. Gilligan, (Ed). Academic Press, London. 231-244 pp.
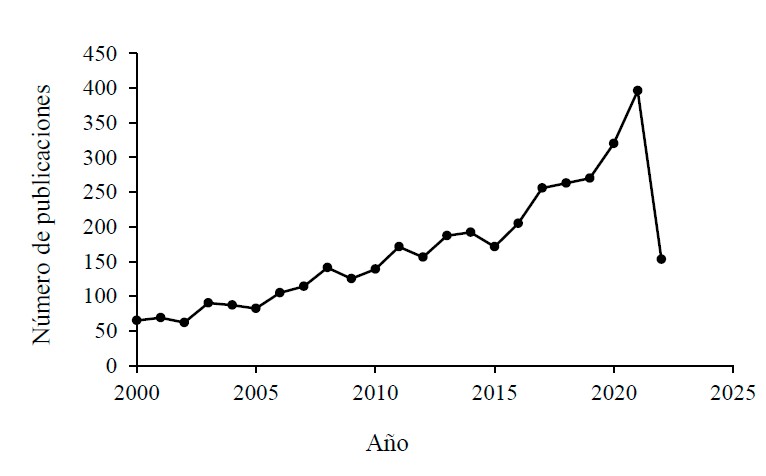
Published
How to Cite
Issue
Section
License
Copyright (c) 2022 Revista Mexicana de Ciencias Agrícolas

This work is licensed under a Creative Commons Attribution-NonCommercial 4.0 International License.
The authors who publish in Revista Mexicana de Ciencias Agrícolas accept the following conditions:
In accordance with copyright laws, Revista Mexicana de Ciencias Agrícolas recognizes and respects the authors’ moral right and ownership of property rights which will be transferred to the journal for dissemination in open access. Invariably, all the authors have to sign a letter of transfer of property rights and of originality of the article to Instituto Nacional de Investigaciones Forestales, Agrícolas y Pecuarias (INIFAP) [National Institute of Forestry, Agricultural and Livestock Research]. The author(s) must pay a fee for the reception of articles before proceeding to editorial review.
All the texts published by Revista Mexicana de Ciencias Agrícolas —with no exception— are distributed under a Creative Commons License Attribution-NonCommercial 4.0 International (CC BY-NC 4.0), which allows third parties to use the publication as long as the work’s authorship and its first publication in this journal are mentioned.
The author(s) can enter into independent and additional contractual agreements for the nonexclusive distribution of the version of the article published in Revista Mexicana de Ciencias Agrícolas (for example include it into an institutional repository or publish it in a book) as long as it is clearly and explicitly indicated that the work was published for the first time in Revista Mexicana de Ciencias Agrícolas.
For all the above, the authors shall send the Letter-transfer of Property Rights for the first publication duly filled in and signed by the author(s). This form must be sent as a PDF file to: revista_atm@yahoo.com.mx; cienciasagricola@inifap.gob.mx; remexca2017@gmail.
This work is licensed under a Creative Commons Attribution-Noncommercial 4.0 International license.